Authorisation
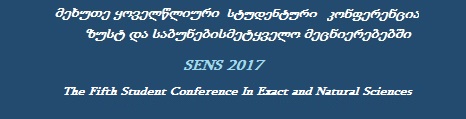
Examining the identification of signals through a neural network
Author: Guram ChaganavaKeywords: Desired signal, Neural network, Multilayer perceptron, Function optimization
Annotation:
This work refers to the problem of identifying and restoring signals. Signal processing is a matter of actual research. The problem is discussed in two different aspects. The first is about the identification of the pre-determined signals from the noisy environment, while second investigates restoring of the distorted, cut signals. Within this research, it is aimed to investigate issue of identification of signals through neural networks. The goal is to study whether it is possible to solve the problem of identification and restoration of signals using intelligent systems. The research considers several methods used in the field of artificial intelligence, popular neural networks and the training algorithms. Construction of appropriate artificial networks is carried out. These networks are tested for each task with different learning parameters. As a result, the optimal configuration of the neural network and the relatively fast optimization algorithm has been selected. Within the first task, it is discussed the process of restoring of the sinusoidal and QPSK modulated signals from different levels of noise. The most optimal results have been achieved through feed-forward deep network, same as multilayer perceptron with Levenberg-Marquardt training algorithm. The network is able to restore the desired signals in a very high precision even when background noise exceeds the signal amplitude several times. As for the second task, the above-mentioned network has managed to use the latter algorithm to recover the cut signal. It is discussed sinusoidal signals with different frequencies and phases which are cut at arbitrary level. As a result, it can be concluded that these problems can be solved by neural networks. It is important that the approach works successfully for signals of different shapes and complexity, indicating that this approach can be generalized for a wide range of signals.